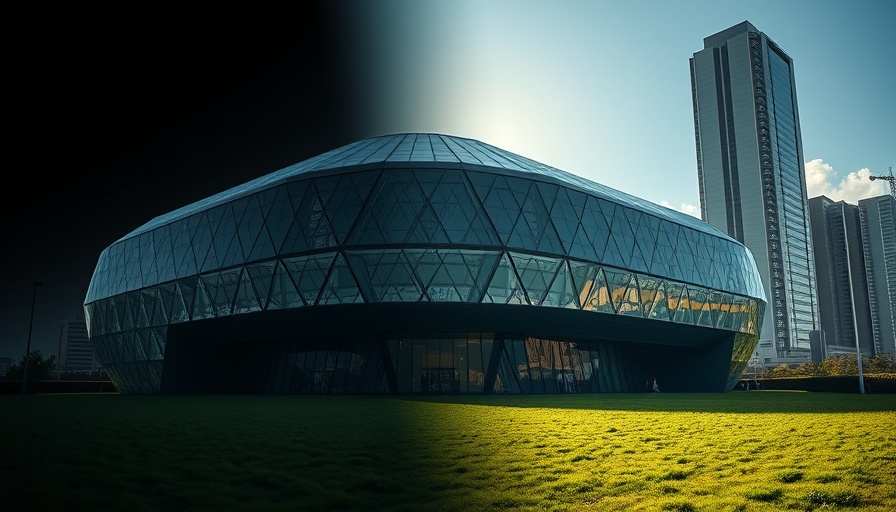
Revolutionizing AI: The Next Step in Diffusion Models
Researchers at the University of California San Diego are pushing the boundaries of machine learning, particularly in the realm of artificial intelligence by enhancing diffusion models—algorithms that generate new content, including images and videos. This innovation is not just about efficiency; it can broaden the scope of what these models can achieve in creative and practical applications.
Understanding Diffusion Models
Diffusion models traditionally enhance learning through a gradual process of adding Gaussian noise to data. This allows the AI to learn complex patterns step by step. However, assistant professor Yian Ma and his team have introduced a novel framework that enables larger jumps between these steps. By considering distributions beyond standard Gaussian noise, such as long-tailed or algorithm-generated distributions, they are streamlining the learning process.
Why This Matters: Implications for AI and Industries
This research, recognized at prestigious machine learning conferences like NeurIPS 2024 and ICML 2024, is set to transform the AI landscape. The ability to accelerate diffusion models can lead to faster development of AI applications across sectors like entertainment, healthcare, and education. Essentially, this means AI can become more responsive and relevant in addressing complex challenges.
Potential Challenges and Considerations
Despite the promising advancements, embracing larger jumps in the learning process isn’t without risks. The use of diverse distributions may introduce unpredictability in the learning outcomes. Researchers must consider these risks while ensuring reliability in model predictions. The balancing act between speed and accuracy is vital for the successful implementation of these technologies.
Looking Ahead: Future Opportunities
The future of diffusion models holds exciting prospects. By accelerating inference, researchers anticipate these models becoming more prevalent in real-time applications. For example, in creative fields, artists could leverage these models to generate complex visuals faster, while medical researchers might use them for modeling and predicting disease patterns effectively.
Conclusion: Embracing Change in AI Technology
The expansion of diffusion models signifies a major leap in machine learning and artificial intelligence. As we stand on the brink of new AI applications, understanding these advancements will help both industry professionals and the general public grasp the implications of faster and more versatile AI technologies. The key will be monitoring how these innovations unfold in practice, ensuring that technological advancements align with ethical considerations.
Write A Comment